What a Future Informed by Data Might Look Like
SafetyCulture News | By | 23 Aug 2018 | 3 minute read
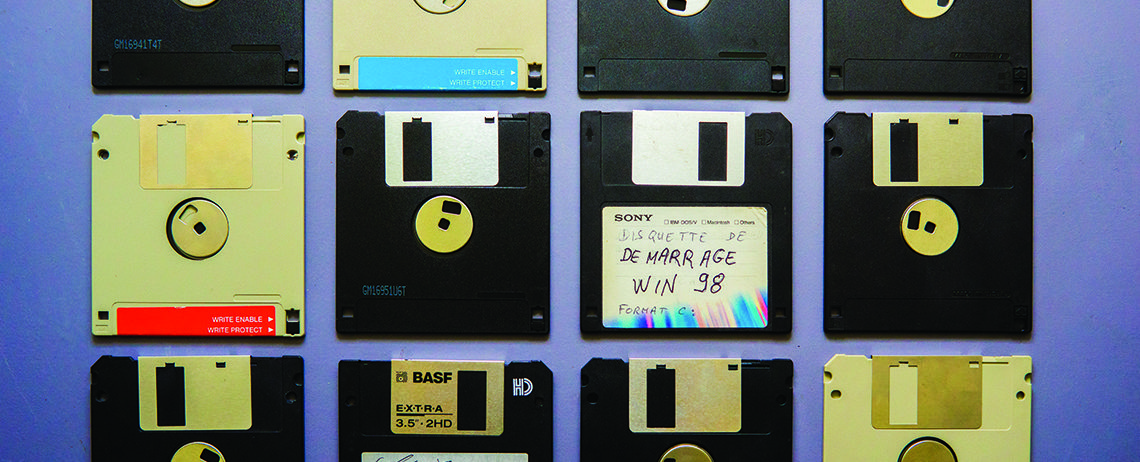
Why are artificial intelligence and machine learning something we care about in checklists?
SafetyCulture’s Tribe Engineering Lead Cameron Newman, who has played a key role in developing iAuditor’s ‘Sites’ feature, imagines what a future informed by data might look like.
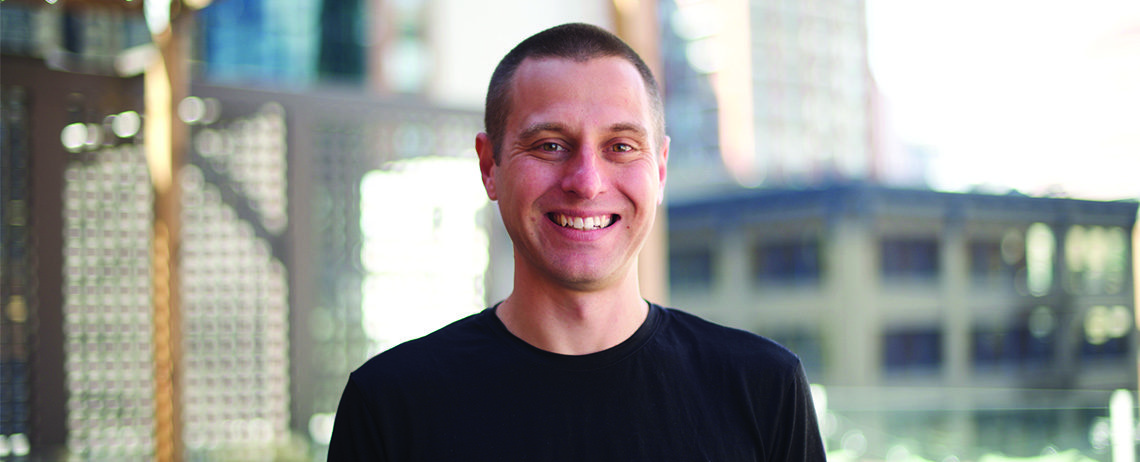
Where do you see machine learning now?
Today at the moment we’re using machine learning (ML) in iAuditor in a very simplified way to suggest or recommend things to frontline workers to enable them to do their job faster and smarter. But we’re working towards a time when artificial intelligence and ML will enable us to actually uncover trends within customer data that would be harder or slower for a human to find.
You can see that already [happening with] where we are using image detection: algorithms are actually trying to detect objects within an image and group them together to then form a thesis around why that’s relevant.
Why is image detection useful for organisations?
We have something like 100 million-plus photos on our system because when customers complete and audit or inspection they’ll often take photos. We want to use ML to run object detection on those images so we can say to a customer, “this particular question in your inspection checklist constantly has images that contain, for example, a bike. Is there a correlation between why that question is failing, based on the objects within it?”
We want to enable them to make better decisions using this data.
Ultimately, we want to get to the point where customers can actually train these algorithms to recognise specific objects within their own business. So, if you’re a mining company and you have specific hardware, we’re not necessarily going to detect that out of our own object library but you can train the app by actually just tagging those images.
What’s the most far-out idea for algorithms?
The next evolution is frontline workers or managers taking a photo, and our models [being able to] say whether the item or object is in a good or a bad state by using machine learning to detect the object and whether it’s in a quality state or a failed state.
We’ve done some research and small projects around taking photos of exposed wires in roofs to train algorithms to identify wires that have a worn sheath and are in a failed state and therefore dangerous. Eventually I expect infrared cameras will be able to pick up this kind of thing faster than humans, which is particularly useful if you’re looking at a complicated object in a difficult or potentially dangerous environment.
Will we get to the point we can open an app and it will say: in three days X object is going to blow up?
Absolutely. Anomaly detection is a big thing. We’re currently looking at integrating Internet of Things technology, where we deploy environmental sensors for things such as temperature and heat or movement. If you can sense and track vibrations within a unit you can normally find or detect an anomaly in it, and it will give you a fairly accurate timeline for when that machine is going to fail, for example if it’s a mechanical bearing issue within the compressor unit.
We saw that in our Townsville office. We put a vibration detector on the air-conditioning unit and you could see visually that it was becoming anomalous in terms of the vibrations cadence and two days later the unit failed.
This anomaly detection is really powerful when you look at data collected by humans, but when you connect it up to sensor data which is reading at every one or five minutes you get a huge volume of data and it’s very easy to detect anomalies in that, and automatically trigger an incident or request and notify the relevant people.
iAuditor’s new in-app feature Sites employs data to make your life easier. Read more about it here.
Important Notice
The information contained in this article is general in nature and you should consider whether the information is appropriate to your specific needs. Legal and other matters referred to in this article are based on our interpretation of laws existing at the time and should not be relied on in place of professional advice. We are not responsible for the content of any site owned by a third party that may be linked to this article. SafetyCulture disclaims all liability (except for any liability which by law cannot be excluded) for any error, inaccuracy, or omission from the information contained in this article, any site linked to this article, and any loss or damage suffered by any person directly or indirectly through relying on this information.